Table Of Content
For instance, relative to some complex interactions, main effects are more easily interpreted (Collins et al., 2014); a factor’s main effects are interpretable even when it interacts with other factors. When effect coding is used, each effect is orthogonal to every other effect in the analysis model (orthogonal when the n’s are equal in each experimental condition, and nearly orthogonal when the n’s differ by a modest amount). Thus, a significant main effect reflects an experimental effect that occurs on average across all other factors in the model even when the relevant factor is involved in significant interactions (Chakraborty et al., 2009).
Lesson 5: Introduction to Factorial Designs
This arises, in part, from the fact that the effects of any given factor are defined by its average over the levels of the other factors in the experiment. It is important, therefore, for researchers to interpret the effects of a factorial experiment with regard to the context of the other experimental factors, their levels and effects. This does not reflect any sort of problem inherent in factorial designs; rather, it reflects the trade-offs to consider when designing factorial experiments.
How to Analyze a 2×2 Factorial Design
For instance, RCTs need not have a true placebo or control condition. Thus, two different active treatments might be contrasted with one another in a two-group design, such as a comparison of two different counseling approaches (e.g., skill training vs. supportive counseling), each paired with the same medication. Neither one of these conditions would be a control condition in a strict sense, since each delivers a different form of active treatment. In addition, an RCT might have a control condition, but this might be used in comparisons with many active treatment conditions.
Analysis
Figure 5.3 shows results for two hypothetical factorial experiments. Time of day (day vs. night) is represented by different locations on the x-axis, and cell phone use (no vs. yes) is represented by different-colored bars. It would also be possible to represent cell phone use on the x-axis and time of day as different-colored bars.
3 - Unreplicated \(2^k\) Factorial Designs
The material found on this site is for informational purposes only and is not intended to be used for diagnosis or treatment purposes. So, the researchers decided to look at how the amount of sleep and caffeine influence test performance. “It is true that when two manipulations are operating simultaneously, it is impossible to disentangle their effects completely,” explain authors Breckler, Olson, and Wiggins in their book Social Psychology Alive.
Factorial Fit: LOGT versus B, C, D
Factorial design-assisted supercritical carbon-dioxide extraction of cytotoxic active principles from Carica papaya leaf ... - Nature.com
Factorial design-assisted supercritical carbon-dioxide extraction of cytotoxic active principles from Carica papaya leaf ....
Posted: Fri, 08 Feb 2019 08:00:00 GMT [source]
And the individual terms, B, C, D, BC and BD, are all significant, just as shown on the normal probability plot above. Notice also the use of the Yates notation here that labels the treatment combinations where the high level for each factor is involved. If only A is high then that combination is labeled with the small letter a. You would find these types of designs used where k is very large or the process, for instance, is very expensive or takes a long time to run. In these cases, for the purpose of saving time or money, we want to run a screening experiment with as few observations as possible. When we introduced this topic we wouldn't have dreamed of running an experiment with only one observation.
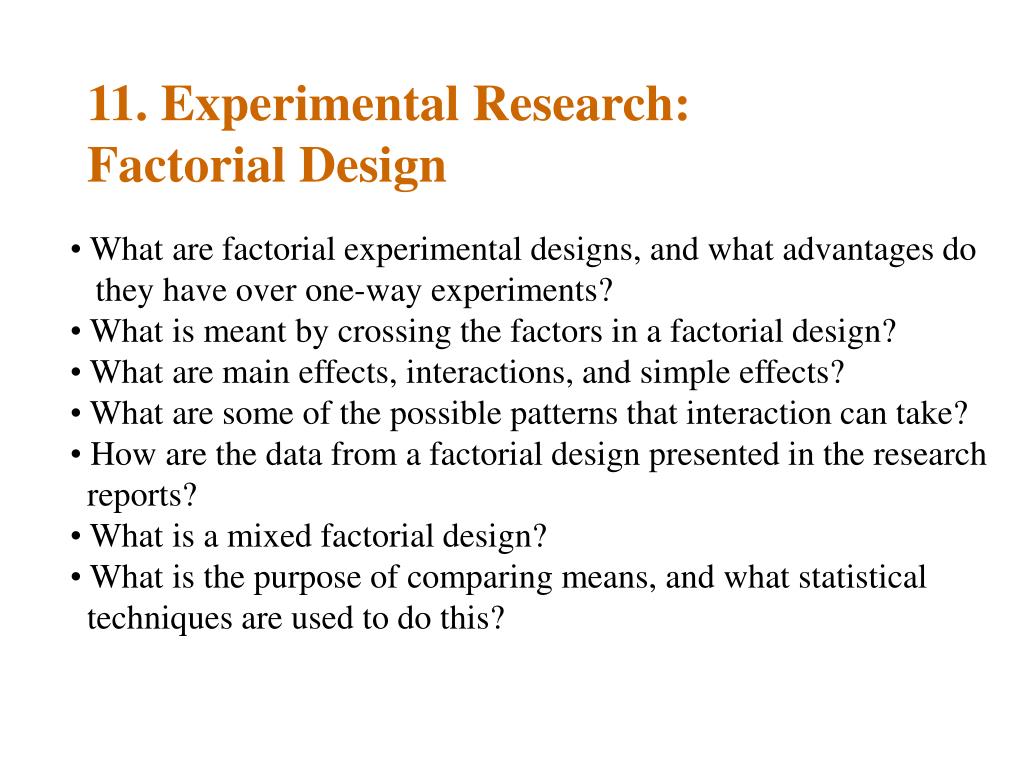
Effects and Interaction Plots
Thus far we have seen that factorial experiments can include manipulated independent variables or a combination of manipulated and non-manipulated independent variables. But factorial designs can also include only non-manipulated independent variables, in which case they are no longer experiments but are instead non-experimental in nature. This can be conceptualized as a 2 × 2 factorial design with mood (positive vs. negative) and self-esteem (high vs. low) as non-manipulated between-subjects factors. But factorial designs can also include only non-manipulated independent variables, in which case they are no longer experiment designs, but are instead non-experimental in nature. But factorial designs can also include only non-manipulated independent variables, in which case they are no longer experiments but are instead non-experimental (cross-sectional) in nature.
Arm Moves to Factor AI into Chip Design with New CPU, GPU - EnterpriseAI
Arm Moves to Factor AI into Chip Design with New CPU, GPU.
Posted: Mon, 29 May 2023 07:00:00 GMT [source]
Selecting Factors: Factor and Intervention Component Compatibility
The advantage of this is that multiple-response measures are generally more reliable than single-response measures. However, it is important to make sure the individual dependent variables are correlated with each other by computing an internal consistency measure such as Cronbach’s \(\alpha\). If they are not correlated with each other, then it does not make sense to combine them into a measure of a single construct. If they have poor internal consistency, then they should be treated as separate dependent variables. A factorial experiment allows for estimation of experimental error in two ways. The experiment can be replicated, or the sparsity-of-effects principle can often be exploited.
In an RCT an “active” treatment arm or condition is statistically contrasted with a “control” treatment arm or condition (Friedman, Furberg, & Demets, 2010). The two conditions should be identical except that the control condition lacks one or more ICs or features that are provided to the active condition. The random assignment of participants to the treatment arms means that the two groups of assigned participants should differ systematically only with regard to exposure to those features that are intentionally withheld from the controls. In smoking cessation research a common RCT design is one in which participants are randomly assigned to either an active pharmacotherapy or to placebo, with both groups also receiving the same counseling intervention. The red bars show the conditions where people wear hats, and the green bars show the conditions where people do not wear hats. For both levels of the wearing shoes variable, the red bars are higher than the green bars.
Or it could be that people who are lower in SES tend to come from certain ethnic groups that emphasize generosity more than other ethnic groups. The researchers dealt with these potential third variables, however, by measuring them and including them in their statistical analyses. They found that neither religiosity nor ethnicity was correlated with generosity and were therefore able to rule them out as third variables. This does not prove that SES causes greater generosity because there could still be other third variables that the researchers did not measure. But by ruling out some of the most plausible third variables, the researchers made a stronger case for SES as the cause of the greater generosity.
You have to be careful about using the mean in one case, and the media in another ... For those of you who have studied heteroscedastic variance patterns in regression models you should be thinking about possible transformations. There is a gap in the histogram of other residuals but it doesn't seem to be a big problem.
Let's use the dataset (Ex6-2.csv) and work at finding a model for this data with Minitab... In the example that was shown above, we did not randomize the runs but kept them in standard order for the purpose of seeing more clearly the order of the runs. In practice, you would want to randomize the order of run when you are designing the experiment. In the example above the A, B and C each are defined by a contrast of the data observation totals. Therefore you can define the contrast AB as the product of the A and B contrasts, the contrast AC by the product of the A and C contrasts, and so forth. One nice feature of the Yates notation is that every column has an equal number of pluses and minuses so these columns are contrasts of the observations.
This is because, as noted earlier, such effects are determined by averaging over the other component effects (with effect coding). In addition, the complexity of delivering multiple combinations of components can be reduced by using a fractional factorial design (Collins et al., 2009), which reduces the number of different component combinations per the number of factors used. While more research on IC interactions is surely needed, our research has consistently found such interactions (Cook et al., 2016; Fraser et al., 2014; Piper et al., 2016; Schlam et al., 2016). Thus, it might be difficult in many cases to assume conditions that would justify the use of a fractional factorial design.
When 40 year olds, however, are given a 5 mg pill or a 10 mg pill, 15% suffer from seizures at both of these dosages. There is an increasing chance of suffering from a seizure at higher doses for 20 year olds, but no difference in suffering from seizures for 40 year olds. Thus, there must be an interaction effect between the dosage of CureAll, and the age of the patient taking the drug. When you have an interaction effect it is impossible to describe your results accurately without mentioning both factors. You can always spot an interaction in the graphs because when there are lines that are not parallel an interaction is present. If you observe the main effect graphs above, you will notice that all of the lines within a graph are parallel.
The figure below contains the DOE table of trials including the two responses. Ignoring the first row, look in the last stage and find the variable that has the largest relative number, then that row indicates the MAIN TOTAL EFFECT. The Main Total Effect can be related to input variables by moving along the row and looking at the first column.
However, by choosing B at the low level you will produce a more homogeneous product, products with less variability. What is important in product manufacturing is not only reducing the number of defects but also producing products that are uniform. This is a secondary consideration that should be taken into account after the primary considerations related to the percent of product defects. For each factor, you see that the residuals are more dispersed (higher variance) to the right than to the left. Overall, however, the residuals do not look too bad and the normal plot also does not look too bad.
No comments:
Post a Comment